SANTA CLARA, Calif. — Nine companies and three universities have launched a research effort to see if machine learning can solve some of the toughest problems in electronics design. The center is one of many efforts across the industry trying to tap into the emerging technology.
Like many ideas in tech, “it all started in a coffee shop one afternoon,” said Elyse Rosenbaum, director of the Center for Advanced Electronics through Machine Learning (CAEML).
“We were facing common problems. We needed behavioral models that interfaced across electro-migration and circuit domains and didn’t know how to go about getting them, given that colleagues were interested in different applications,” Rosenbaum said in a panel on the topic at the DesignCon event here.
“We knew we would get no funding for one specific problem, so we decided we needed to solve them all, reaching out to other universities to work together to investigate different machine-learning techniques and algorithms that are well suited to use in electronics,” she said.
The work got backing from the National Science Foundation as well as nine companies: Analog Devices, Cadence, Cisco, Hewlett-Packard Enterprise (HPE), IBM, Nvidia, Qualcomm, Samsung, and Xilinx. The center is jointly hosted at the University of Illinois Urbana-Champaign, North Carolina State University (NCSU), and Georgia Tech.
So far, the group has identified interest areas that include high-speed interconnects, power delivery, system-level electrostatic discharge (ESD), IP core reuse, and design rule checking. Rosenbaum’s research team will explore use of recurrent neural nets to model ESD characteristics of circuits so that systems pass qualification tests the first time.
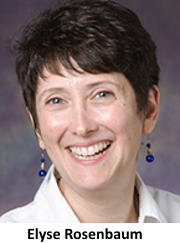
“We would like to model phenomena that we can’t using existing techniques … such as ESD characteristics that depend on a power-delivery network and multicore interactions” in a processor, she said.
One of the hurdles is finding ways to limit neural net predictions to physically valid outputs. Overall, researchers need to carefully construct each step in the machine-learning process from acquiring good training data to selecting candidate models, training them, and validating their results, she said.
“Most of what we usually create are discriminative models with expected outputs, [but machine learning creates] generative models [that] give probabilities between inputs and outputs — this is useful for statistical issues like manufacturing variances in chips,” she added.
Chris Cheng, a distinguished technologist in HPE’s storage division, gave several examples of areas where he would like to apply machine learning. For example, he foresees chip vendors in the future providing interactive component models as neural nets engineers can test and train over cloud services.
Cheng also suggested that channel analysis could be handled as a cloud service using machine learning. In addition, he sketched out an idea for embedding neural nets in an oscilloscope that dynamically learns equalization techniques.
Cadence is already trying to leverage machine learning to break through knotty problems in chip design, said David White, a senior group director for R&D on the company’s Virtuoso analog design tool. Machine learning could provide ways to handle the increase in design rules and large chip designs at advanced nodes.
White described a future tool that could provide feedback during the course of a design on issues such as electro-migration and parasitic extraction. Such a capability could reduce the many iterative loops that chip designers cycle through today, he said.
Paul Franson, a professor at NCSU, said that one student has already used machine learning to reduce iterations in chip routing from 20 to four.
— Rick Merritt, Silicon Valley Bureau Chief, EE Times 
Related posts:
- DesignCon 2017 Bets Big on Power Management
- Baidu Releases AI Benchmark
- Machine Learning Routes Chips
- System-in-Package Gets 100G Link